Mapping the Landscape of AI-Powered Nonprofits
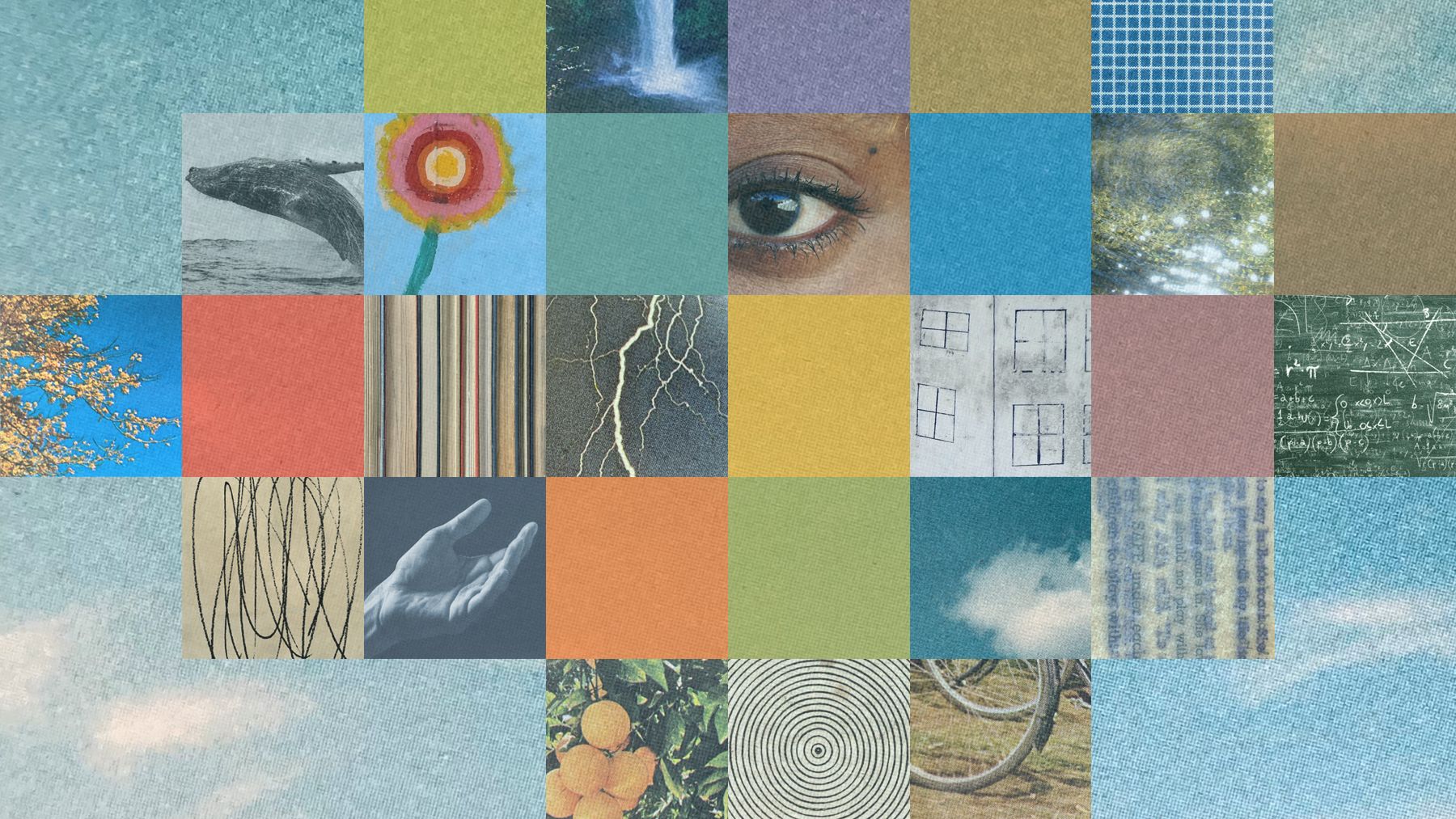
Originally published in Stanford Social Innovation Review by Kevin Barenblat and Brigitte Hoyer Gosselink.
Visualize the year 2050. How do you see AI having impacted the world? Whatever you’re picturing… the reality will probably be quite a bit different. Just think about the personal computer. In its early days circa the 1980s, tech companies marketed the devices for the best use cases they could imagine: reducing paperwork, doing math, and keeping track of forgettable things like birthdays and recipes. It was impossible to imagine that decades later, the larger-than-a-toaster-sized devices would be smaller than the size of Pop-Tarts, connect with billions of other devices, and respond to voice and touch.
It can be hard for us to see how new technologies will ultimately be used. The same is true of artificial intelligence. With new use cases popping up every day, we are early in the age of AI. To make sense of all the action, many landscapes have been published to organize the tech stacks and private sector applications of AI. We could not, however, find an overview of how nonprofits are using AI for impact.
We wanted to understand exactly what was happening in the emerging “AI-powered nonprofit” space. Why? First, it’s tough to build what you can’t see. Providing a landscape helps other social entrepreneurs see—and get inspired by—the vast possibilities in applying AI for social good. Second, we want to help demystify the space for funders, so they can help actualize the potential of this new tech to drive impact.
AI-powered nonprofits (APNs) are already advancing solutions to many social problems, and Google.org’s recent research brief AI in Action: Accelerating Progress Towards the Sustainable Development Goals shows that AI is driving progress towards all 17 SDGs. Three goals that stand out with especially strong potential to be transformed by AI are SDG 3 (Good Health and Well-Being), SDG 4 (Quality Education), and SDG 13 (Climate Action). As such, this series focuses on how AI-powered nonprofits are transforming the climate, health care, and education sectors.
Just as the private AI sector is growing at unprecedented rates, so too is the APN sector. Collaborative Google.org research found a 300 percent increase in the application of AI to address the SDGs since 2018. What’s more, the most recent applicant pool for Fast Forward’s Startup Accelerator, a three-month program for early-stage nonprofit startups, saw a 600 percent increase in AI-powered nonprofit applicants compared to the year prior. New solutions are coming online every week.
To make sense of it all, Fast Forward analyzed nearly 100 use cases, collaborated with leaders in the tech-for-good ecosystem, and interviewed dozens of builders. These conversations helped us classify what we see happening. We developed a working landscape to connect the dots, and in the end, four big categories of nonprofit AI use cases emerged: Structuring Data, Advising, Translating, and Platforms. Excitingly, we found that most APNs don’t use AI in just one way. Their solutions tend to consist of a constellation of use cases.
We’ll dig in to each of these categories, but first a few special considerations around the creation of our landscape:
- This landscape focuses on AI to advance core impact objectives. It does not wade into how AI can improve nonprofit internal operations. More on that later.
- This ontology is by no means exhaustive. By the time you read this article, we will likely have discovered new and different ways nonprofits are using AI.
- The use cases are sector-agnostic and can be applied across every issue area, from education to human rights to climate.
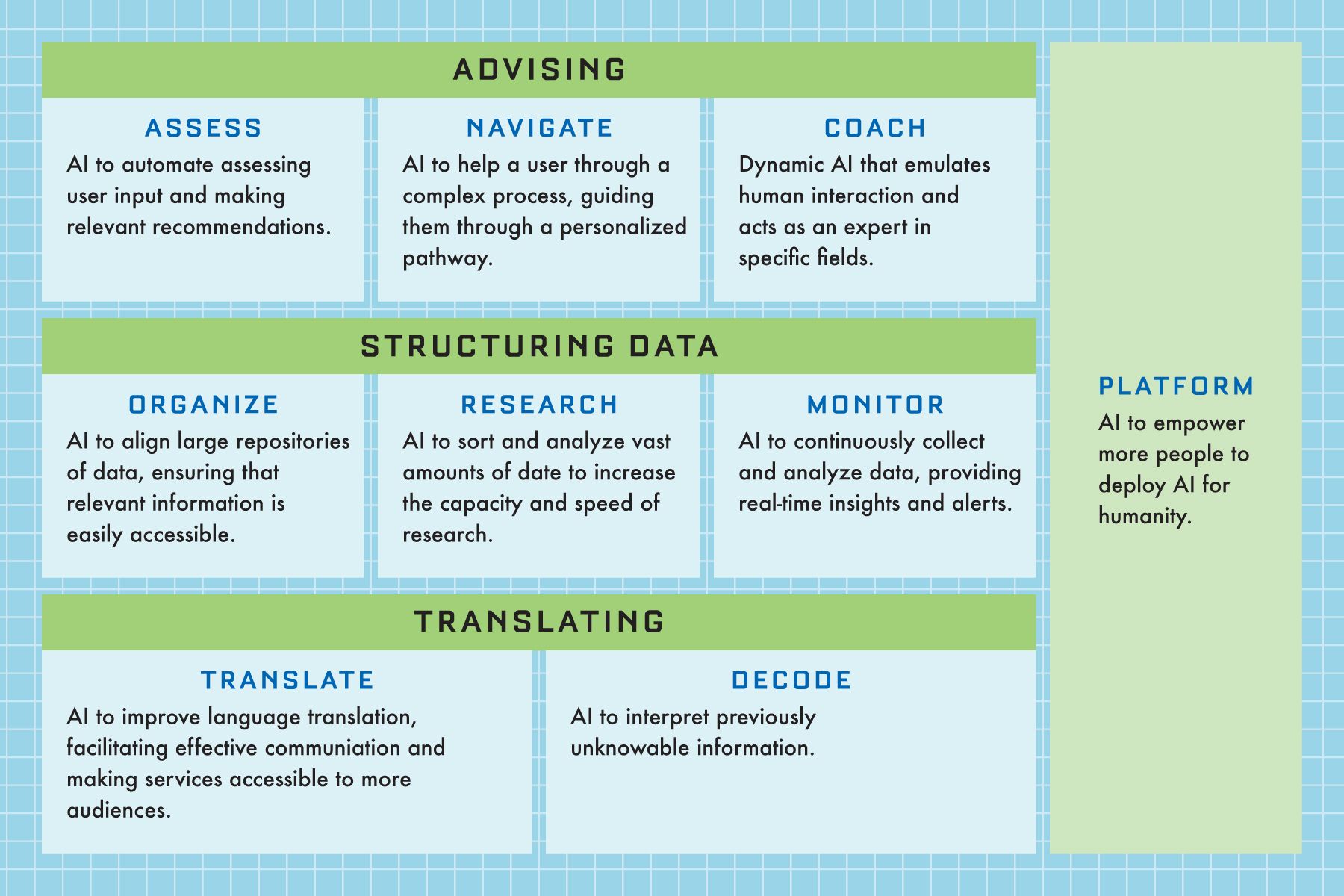
The Growing Landscape of AI-Powered Nonprofits
Structuring Data
AI solutions in this category supercharge what humans can do with data. Before access to AI tools became widespread, an organization needed a data scientist to make sense of big, unstructured datasets. We used to learn the language of the machine. Now machines, through the consumer-facing interfaces enabled by generative AI, can respond to our language. The advent of accessible AI tools means that not-that-technical people can make sense of—and interact with—data. Within this category, Fast Forward found three major sub-branches:
- Research: AI to sort and analyze vast amounts of data to increase the power and speed of research. Research tasks that used to take years are now possible in a matter of days, and by a single researcher rather than a team. RebootRx, for example, uses AI to sort through hundreds of thousands of research papers to identify generic drugs suitable for cancer treatment.
- Organize: AI to align large corpora of data, ensuring that relevant information is easily accessible. Many nonprofits can say goodbye to week-long marathons of sorting through spreadsheets or reams of documents. Learning Equality is a global education nonprofit that aligns large libraries of education content to a given country’s curriculum standards. For example, in Uganda, they matched 12,000 learning resources with more than 2,000 learning categories—a herculean task that would take months without AI.
- Monitor: AI to continuously collect and analyze data, providing real-time insights and alerts. Complex data gathered from sensors all across the world can be combined and transformed to generate simple, human-interpretable insights and clear recommended actions. We love WattTime as an example. The nonprofit, as part of the Climate TRACE coalition, uses AI to monitor vast amounts of satellite images to reveal greenhouse gas emissions of big polluters.
Advising
This category is AI deployed to scale human-to-human experiences. It is the user-facing AI category that many of us encounter every day. Think of chatbots like Gemini, ChatGPT, Midjourney, etc. What makes AI-powered nonprofits’ contributions in this category unique is that their products are designed for extremely specific audiences who may otherwise be overlooked. Given the vulnerable populations that many of these APNs are built for, considerations around user experience and safety are paramount.
Advising is comprised of three sub-branches:
- Assess: AI to automate assessing user input and making relevant recommendations. This type of AI provides tailored recommendations based on user inputs, with the interaction process designed to be straightforward and uniform. Joy Education exemplifies this. Young readers read to an AI-enabled-friend for three minutes. The AI diagnoses the reader’s areas for growth and then provides teachers and parents with a customized learning plan.
- Navigate: AI to help a user through a complex process, guiding them through a personalized pathway. This use case is applicable for complicated, multistep processes like applying for financial aid or navigating a new health-care system. For example, HERA Digital Health provides refugees personalized, step-by-step health-care guidance during emergencies.
- Coach: Dynamic AI that emulates human interaction and acts as an expert in specific fields. With these tools, communities gain immediate access to high-level support without having to pay a professional. Khanmigo, Khan Academy’s free, AI-powered teaching assistant, aims to offer subject matter experts to all students. In this way, generative AI is also being used to create curriculum for teachers, support agriculture workers, and empower community health workers. In other words: helping the helpers.
Translating
This category is AI to ... translate! Good translation is key to increasing access. We’re seeing AI for translation deployed across sectors, unlocking opportunities all over the globe. And it’s more than just translating language. With GenAI, nonprofits can bridge contexts too. This especially matters for nonprofits that work across communities, cultures, contexts—and even species.
We’re seeing translating break down into two sub-branches:
- Translate: AI to improve language translation, facilitating effective communication and making services accessible to more audiences. Because translation is typically a manual and time-intensive task, it can be quite expensive, especially for less widely spoken languages. Moreover, translating, even to major languages, can be unrealistic for cash-strapped social service providers. The scale and economy of AI-powered translation can help remove these language barriers. U.S. Digital Response, as one example, uses AI to power its unemployment insurance tool, which provides plain-language Spanish translations for workers with limited English proficiency.
- Decode: AI to interpret previously incomprehensible information. Understanding what animals are saying, for example, feels like a capability limited to our wildest dreams. But machines can pick up information and patterns that humans can’t. For instance, Earth Species Project uses deep learning to visualize semantic representations of language to unlock communication with other species.
Platform
This type of AI empowers more nonprofits to deploy their own AI tools. Think of customizable chatbots or open-source platforms. Rather than delivering pre-packaged solutions, these nonprofits empower people to customize technology to meet their own needs. For example, Playlab provides educators with AI tools to create, remix, and share their own, customizable apps.
“We believe that the benefits of these new AI technologies cannot remain locked and accessible to only a few. We must work to ensure everyone benefits equally from them.”
- Gemma Turon, PhD, Co-founder of Ersilia
What if nonprofits can’t find a way their programs could benefit from any of these AI use cases? Or if they don’t have a CTO or the tech talent to implement it? They may still be able to create value by deploying AI for internal operations. It can give workers and leaders time back so they can focus more of their energy on mission-critical work. A recent Google.org report suggests organizations can get started by seeing where they can leverage AI in a couple of main ways. First, they can use AI to create personalized marketing campaigns that are engaging, informative, and more likely to resonate with potential donors. Next is fundraising. An easy introduction is to try automating repetitive tasks, like fitting messages into a certain word count and customizing emails.
When used effectively, AI is turbocharging nonprofits’ ability to serve their beneficiaries. The ability to provide thoughtful, niche support at any time of day, organize data at record speed, and converse with people who speak a different language can change the game for nonprofits.
Founders, funders, and believers in AI for good need to bring our very best tools to the table to solve the scale of problems we face today. We also need to be intentional about how we conceptualize AI for social good: AI is a means, not the end. An AI-powered product is certainly not a silver bullet for solving a social problem. Nonprofits must remain impact first, deploying AI only where it can help nonprofits scale. In addition, cross-sector partnerships, for example between on-the-ground nonprofits and AI-powered nonprofits, can be a powerful boon in addressing pressing societal challenges. And of course, a commitment to responsible deployment is non-negotiable when deploying AI for social impact. Considerations around safe usage, data privacy, and avoiding harmful bias are especially important when working with vulnerable populations.
In the words of Gemma Turon, PhD, co-founder of APN Ersilia, “We believe that the benefits of these new AI technologies cannot remain locked and accessible to only a few. We must work to ensure everyone benefits equally from them.” Technological advancement has historically overlooked the needs of the most vulnerable. Nonprofits and civil society belong at the forefront of AI. By bolstering communities’ agency to decide when and how to use it, the transformation can happen with them, not to them.
In the articles to come, we will dive deep into use cases across sectors: climate, health care, and education. This will give a look into the many ways AI is being used to serve humanity for the better.
Nevertheless, sometime soon, these use cases might seem archaic. We are skeuomorphic, in that the first step in technological innovation is typically replacing current use cases. But it is the next step that can change paradigms. The social sector will soon deliver even bigger impacts in ways we haven’t yet imagined.